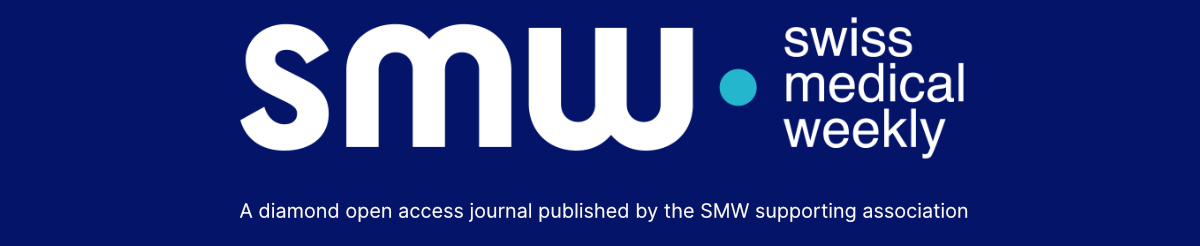
With the emerging advances made in genomics and functional genomics approaches, there is a critical and growing unmet need to integrate plural datasets in order to identify driver genes in cancer. An integrative approach, with the convergence of multiple types of genetic evidence, can limit false positives through a posterior filtering strategy and reduce the need for multiple hypothesis testing to identify true cancer vulnerabilities. We performed a pooled shRNA screen against 906 human genes in the oral cancer cell line AW13516 in triplicate. The genes that were depleted in the screen were integrated with copy number alteration and gene expression data and ranked based on ROAST analysis, using an integrative scoring system, DepRanker, to compute a Rank Impact Score (RIS) for each gene. The RIS-based ranking of candidate driver genes was used to identify the putative oncogenes AURKB and TK1 as essential for oral cancer cell proliferation. We validated the findings, showing that shRNA mediated genetic knockdown of TK1 or pharmacological inhibition of AURKB by AZD-1152 HQPA in AW13516 cells could significantly impede their proliferation. Next we analysed alterations in AURKB and TK1 genes in head and neck cancer and their association with prognosis using data on 528 patients obtained from TCGA. Patients harbouring alterations in AURKB and TK1 genes were associated with poor survival. To summarise, we present DepRanker as a simple yet robust package with no third-party dependencies for the identification of potential driver genes from a pooled shRNA functional genomic screen by integrating results from RNAi screens with gene expression and copy number data. Using DepRanker, we identify AURKB and TK1 as potential therapeutic targets in oral cancer. DepRanker is in the public domain and available for download at http://www.actrec.gov.in/pi-webpages/AmitDutt/DepRanker/DepRanker.html.