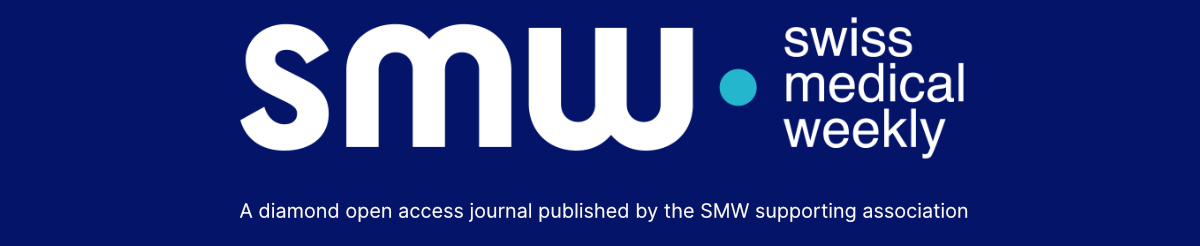
BACKGROUND: Improvement of paediatric healthcare is hampered by inefficient processes for generating new evidence. Clinical research often requires extra encounters with patients, is costly, takes place in an artificial situation with a biased selection of patients, and entails long delays until new evidence is implemented into health care. Electronic health records (EHR) contain detailed information on real patients and cover the entirety of patients. However, the use of EHR for research is limited because they are not standardised between hospitals. This leads to disproportionate amounts of work for extracting data of interest and frequently data are incomplete and of poor quality.
AIMS: SwissPedData aims to lay the foundation for a paediatric learning health system in Switzerland by facilitating EHR-based research. In this project, we aimed to assess the way routine clinical data are currently recorded in large paediatric clinics in Switzerland and to develop a national EHR-based set of common data elements (CDEs) that covers all processes of routine paediatric care in hospitals.
METHODS: A taskforce of paediatricians from large Swiss children’s hospitals reviewed the current status of routine data documentation in paediatric clinical care and the extent of digitalisation. We then used a modified Delphi method to reach a broad consensus on a national EHR-based set of CDEs.
RESULTS: All Swiss children’s hospitals use EHR to document some or all aspects of care. One hundred and nineteen paediatricians, representing eight hospitals and all paediatric subspecialties, participated in an extended Delphi process to create SwissPedData. The group agreed on a national set of CDEs that comprises a main module with general paediatric data and sub-modules relevant to paediatric subspecialties. The data dictionary includes 336 CDEs: 76 in the main module on general paediatrics and between 11 and 59 CDEs per subspecialty module. Among these, 266 were classified as mandatory, 52 as recommended and 18 as optional.
CONCLUSION: SwissPedData is a set of CDEs for information to be collected in EHR of Swiss children’s hospitals. It covers all care processes including clinical and paraclinical assessment, diagnosis, treatment, disposition and care site. All participating hospitals agreed to implement SwissPedData in their clinical routine and clinic information systems. This will pave the way for a national paediatric learning health system in Switzerland that enables fast and efficient answers to urgent clinical questions by facilitating high-quality nationwide retrospective and prospective observational studies and recruitment of patients for nested prospective studies and clinical trials.