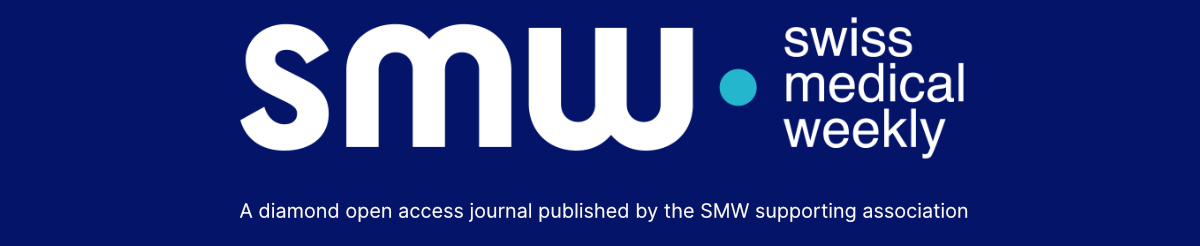
The reproduction number is broadly considered as a key indicator for the spreading of the COVID-19 pandemic. Its estimated value is a measure of the necessity and, eventually, effectiveness of interventions imposed in various countries. Here we present an online tool for the data-driven inference and quantification of uncertainties for the reproduction number, as well as the time points of interventions for 51 European countries. The study relied on the Bayesian calibration of the SIR model with data from reported daily infections from these countries. The model fitted the data, for most countries, without individual tuning of parameters. We also compared the results of SIR and SEIR models, which give different estimates of the reproduction number, and provided an analytical relationship between the respective numbers. We deployed a Bayesian inference framework with efficient sampling algorithms, to present a publicly available graphical user interface (https://cse-lab.ethz.ch/coronavirus) that allows the user to assess and compare predictions for pairs of European countries. The results quantified the rate of the disease’s spread before and after interventions, and provided a metric for the effectiveness of non-pharmaceutical interventions in different countries. They also indicated how geographic proximity and the times of interventions affected the progression of the epidemic.