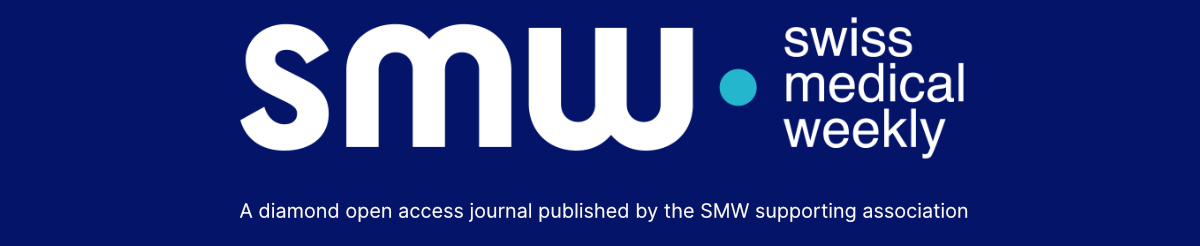
Following the rapid dissemination of COVID-19 cases in Switzerland, large-scale non-pharmaceutical interventions (NPIs) were implemented by the cantons and the federal government between 28 February and 20 March 2020. Estimates of the impact of these interventions on SARS-CoV-2 transmission are critical for decision making in this and future outbreaks. We here aim to assess the impact of these NPIs on disease transmission by estimating changes in the basic reproduction number (R0) at national and cantonal levels in relation to the timing of these NPIs. We estimated the time-varying R0 nationally and in eleven cantons by fitting a stochastic transmission model explicitly simulating within-hospital dynamics. We used individual-level data from more than 1000 hospitalised patients in Switzerland and public daily reports of hospitalisations and deaths. We estimated the national R0 to be 2.8 (95% confidence interval 2.1–3.8) at the beginning of the epidemic. Starting from around 7 March, we found a strong reduction in time-varying R0 with a 86% median decrease (95% quantile range [QR] 79–90%) to a value of 0.40 (95% QR 0.3–0.58) in the period of 29 March to 5 April. At the cantonal level, R0 decreased over the course of the epidemic between 53% and 92%. Reductions in time-varying R0 were synchronous with changes in mobility patterns as estimated through smartphone activity, which started before the official implementation of NPIs. We inferred that most of the reduction of transmission is attributable to behavioural changes as opposed to natural immunity, the latter accounting for only about 4% of the total reduction in effective transmission. As Switzerland considers relaxing some of the restrictions of social mixing, current estimates of time-varying R0 well below one are promising. However, as of 24 April 2020, at least 96% (95% QR 95.7–96.4%) of the Swiss population remains susceptible to SARS-CoV-2. These results warrant a cautious relaxation of social distance practices and close monitoring of changes in both the basic and effective reproduction numbers.