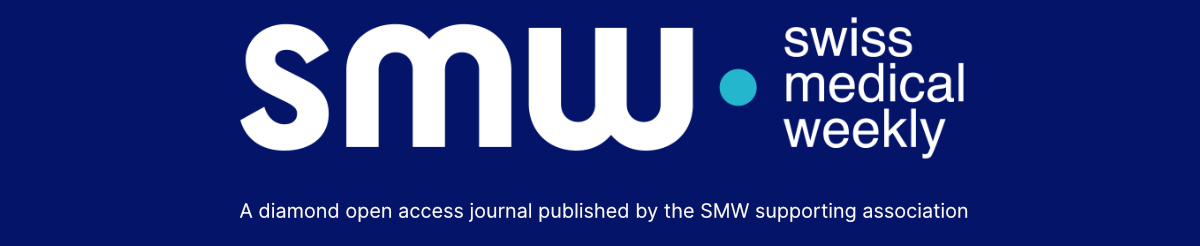
Technological advances in the ability to read the human genome have accelerated the speed of sequencing, such that today we can perform whole genome sequencing (WGS) in one day. Until recently, genomic studies have largely been limited to seeking novel scientific discoveries. The application of new insights gained through cancer WGS into the clinical domain, have been relatively limited. Looking ahead, a vast amount of data can be generated by genomic studies. Of note, excellent organisation of genomic and clinical data permits the application of machine-learning methods which can lead to the development of clinical algorithms that could assist future clinicians and genomicists in the analysis and interpretation of individual cancer genomes. Here, we describe what can be gleaned from holistic whole cancer genome profiling and argue that we must build the infrastructure and educational frameworks to support the modern clinical genomicist to prepare for a future where WGS will be the norm.